Are the solar industry’s pre-construction production estimates too optimistic? Yes, according to several contributions to the “Solar Risk Assessment: 2020” paper published by kWh Analytics. kWh found evidence of “an industry wide bias towards aggressive predictions,” noting that “when a typical solar project performs at the official “P90,” equity cash yield drops by 50%.” DNV GL found optimistic irradiance assumptions contributing to underperformance in the range of 2% of P50 revenue.
There are a few ways to select solar resource data, some better than others. We recommend a simple and tested approach: use a high-quality data service such as SolarAnywhere®. Project stakeholders using SolarAnywhere can feel confident in their predictions. And to avoid any doubt, there is no evidence of a high bias in SolarAnywhere Data.
An alternative approach to solar resource data selection—call it the median approach—has emerged as a potential remedy to optimistic irradiance assumptions. In the median approach, many typical year datasets are compared (the more the better), and the one with the median GHI is selected. While comparing datasets can be informative, the median approach to solar resource data selection falls short. Let’s briefly explore three issues with the median approach:
- The median approach lacks spatial precision
- The median approach does not resolve biases and issues with lower quality datasets
- The median approach lacks transparency and repeatability
Issue 1: The median approach lacks spatial precision
Most of the data available for the median approach are for legacy TMY2/TMY3 locations. Few if any of these locations are likely to be co-located with your project, so the readings may not be representative of the solar resource there.
In addition, taking the median of data for various locations artificially smooths gradients in the solar resource. You can prove this to yourself by testing the median approach anywhere on the island of Oahu, Hawaii. If you get ~5.35 kWh/m^2/day (annual average 223 W/m^2), that’s because most of the data comprising the median is the same regardless of the location you selected. In reality, we know that weather on the island can vary substantially within a couple of kilometers. This is illustrated by the map of enhanced resolution SolarAnywhere Data in Figure 1.
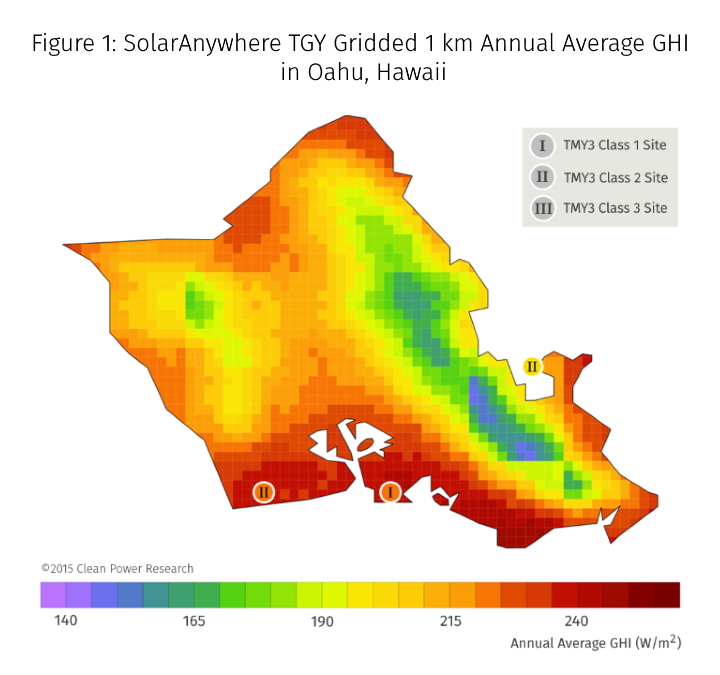
Issue 2: The median approach does not resolve biases and issues with lower quality datasets
The median approach is heavily weighted with lower quality data including legacy data from NREL (TMY2/TMY3) and less accurate satellite models (e.g. NREL PSM). We wrote about the perils of using outdated datasets like TMY3 in 2015. Spatial resolution, inconsistent methodology, legacy measurement techniques and older periods contribute to the differences. At the end of the day, TMY3 was never meant for solar project financing (as has been stated by NREL). The data is based on modeling and measurements from 1976-2005. In addition, TMY3 is only 50% weighted for irradiance; the other 50% includes wind speed, temperature and dew point, which have a much smaller impact on solar production.
Other satellite models like NREL’s PSM have not been vetted for use in solar project financing. The biases in the PSM are well documented.1
Does taking the median of lower quality data yield a bankable result? An analysis of 14 locations using the highest quality SURFRAD/SOLRAD2 measurements as a reference shows that it does not. The median approach does not resolve biases in the underlying datasets. What’s more, using SolarAnywhere alone substantially reduces production risk when compared to the median approach and legacy datasets. If you’d like to review the data for yourself, please contact us.
Issue 3: The median approach lacks transparency and repeatability
The median approach to solar resource selection is not supported by published validation (prompting us to perform this analysis). Repeating the median approach requires keeping track of the input datasets. If one dataset is swapped or removed, the median changes. The process for selecting the datasets for inclusion in the median is left up for debate.
Finally, the median TMY isn’t comparable to recent ground measurements from the project site because the TMY lacks recent data for comparison. If ground measurements are available pre-construction, its apples to oranges with the TMY. Once the plant comes online, performance engineers will have a difficult time distinguishing plant performance and pro forma expectations from recent weather.
All these issues make it difficult for project stakeholders to make efficient and objective decisions.
By contrast, SolarAnywhere Data has extensive public validation with known uncertainty, data versioning and consistent data historical through real time to list a few of the five factors defining bankable solar resource data.
Conclusions: the source of solar resource data matters
The median approach to resource selection can both over- and underestimate the true solar resource depending on the specifics of the project and the input data used. The median approach lacks spatial precision, and does not resolve biases in lesser quality datasets used as input. In addition, the median approach lacks the transparency and repeatability required for objective and efficient decision-making.
While comparing datasets can be informative, in general, using SolarAnywhere alone gives substantially better results than the median approach to solar resource data selection. To see more data on this topic, or to learn more about our trusted solar data and intelligence services, please contact us.
2BSRN: Driemel, A., Augustine, J., Behrens, K., Colle, S., Cox, C., Cuevas-Agulló, E., Denn, F. M., Duprat, T., Fukuda, M., Grobe, H., Haeffelin, M., Hodges, G., Hyett, N., Ijima, O., Kallis, A., Knap, W., Kustov, V., Long, C. N., Longenecker, D., Lupi, A., Maturilli, M., Mimouni, M., Ntsangwane, L., Ogihara, H., Olano, X., Olefs, M., Omori, M., Passamani, L., Pereira, E. B., Schmithüsen, H., Schumacher, S., Sieger, R., Tamlyn, J., Vogt, R., Vuilleumier, L., Xia, X., Ohmura, A., and König-Langlo, G.: Baseline Surface Radiation Network (BSRN): structure and data description (1992–2017), Earth Syst. Sci. Data, 10, 1491-1501, doi:10.5194/essd-10-1491-2018, 2018.