Introduction
Accumulated snow can reduce the energy output of a solar PV system by obstructing the sunlight available for energy conversion. PV energy losses due to snow—commonly referred to as “snow losses”—can vary widely between locations and time periods.
Estimating snow losses accurately can help PV system owners and financiers reduce financial and operational risk to their solar projects. However, several factors can impact the magnitude of snow losses. The local climate, as well as the PV system’s configuration (such as the tilt and tracking type) can determine the rate of snow accumulation and shedding. Additionally, since the local weather is always in flux—changing from season to season and year to year—snow losses can exhibit high seasonal and inter-annual variability.
By incorporating the full-time series weather data at a project location with a snow loss model, customers can more accurately predict the impact of snow losses on PV energy output.
Modeling snow losses with SolarAnywhere
SolarAnywhere energy modeling services support the pvlib snow loss model developed by NREL (referred henceforth as ‘NREL snow loss model’) and Townsend.1,2,5 The NREL model estimates the fraction of the PV row slant height that is covered by snow at each time step. SolarAnywhere time-series snow-depth data is used to determine the accumulated snowfall in each time step. If the snowfall exceeds the threshold, the PV modules are considered to be fully covered by snow.3 The NREL model considers snow sliding as the dominant process of snow removal and does not account for the effect of snow melting or wind removal.2 The Townsend snow loss model uses SolarAnywhere time-series snow-depth data to determine monthly snow totals and snow events. The Townsend model determines the percentage of lost DC capacity due to snow coverage and ambient conditions.5
The NREL snow-loss model validation is primarily focused on fixed-tilt systems. It was found that incorporating the snow loss model improved the accuracy of estimated annual energy production for the PV systems tested. The model was also found to reduce errors for a single-axis tracking system. A comparison of the NREL snow loss model (referred to as Marion Snow Loss Model in Figure 1, below) and Townsend models with other snow loss models is shown in Figure 1.4
Figure 1: Comparison of Snow Loss Models4
Source: SolarPro Magazine: Modeling Losses Due to Snowfall
Snow loss modeling is supported in the SolarAnywhere API and is available with historical and real-time time-series data only. Real-time time-series requests for snow loss output fields outside the Continental United States are limited to the trailing week rather than the current hour. Because snow loss calculations are based on accumulated snow, SolarAnywhere loss modeling considers snow depth and ambient temperature data for six months prior to the start time specified in your simulation request.
Using time-series weather data to make snow-loss estimates more site specific
With SolarAnywhere Sites , customers can access full historical time-series weather data at their project site. Detailed information about daily weather patterns such as snow depth and ambient temperature can be leveraged by energy simulation tools to generate site-specific loss estimates.
To understand how snow loss estimates can be made more accurate, we compared two approaches:
- A “Generic Loss Approach” that uses loss estimates provided in an NREL study of the PV snow loss model in the System Advisor Model (SAM)
- A “Specific Loss Approach” that incorporates SolarAnywhere time-series data with the snow loss model in SAM
Key differences between the two approaches are listed in Figure 2. Both approaches use NREL’s Marion model to estimate snow losses. The key difference is in the weather data period of record and the snow data spatial resolution.
For example, the generic approach uses NSRDB weather data for the period 1961-1990. This is a relatively older period of record compared to the specific approach, which uses more recent time-series weather data. Another key difference is the spatial resolution. The snow data used in the generic approach is interpolated from 239 locations across the United States. Compared to this approach, the specific approach uses satellite derived meteorological data at a 4 km spatial resolution.
Figure 2: Comparison of Generic Loss Approach with Specific Loss Approach
Annual Snow Losses
We modeled annual snow losses for an arbitrary utility-scale PV system (50 MWdc, DC:AC ratio 1.3) with a 20-degree fixed-tilt. The system was placed across five different locations in the U.S. Figure 3 shows that the Generic Loss Approach can result in both over- or under-estimation of snow losses at an energy site.
So what does this mean for PV energy estimates? As shown in Figure 4, the difference in annual energy yield using the Generic and Specific Loss Approaches can easily reach 7-8%, and this can further vary from year to year and location to location.
Figure 4: Difference in Annual Energy Yield Using Generic and Specific Loss Approaches for the Year 2020
The system configuration (such as tracking type and tilt) can further affect the magnitude of energy losses. To demonstrate this, NREL performed a study comparing five different system designs at an energy site in Colorado. As shown in Figure 5, energy losses can easily exceed 9% for a low-tilt, fixed PV system.
Calculating P50/P90 snow loss estimates
Since snow losses are cumulative and time-dependent in nature, using multiple years of time-series data can improve the reliability and accuracy of loss estimates, and account for the effect of interannual variability in snow losses. To demonstrate how P50/P90 estimates can be made more data driven and site specific with more high-fidelity and up-to-date time-series data, we calculated P50/P90 snow loss estimates using SolarAnyhwere historical time-series weather data from 2004-2020 in SAM and compared them with generic estimates. An arbitrary utility-scale PV system was placed across five different locations in the U.S. Two energy site configurations were considered: a 20-degree fixed-tilt and a single-axis tracking PV system.
Figure 6 shows how the P50/P90 estimates can be made more data driven and site specific with time-series modeling compared to generic estimates. Often, financing terms may be set on downside annual energy production (e.g., a P90 or P99 estimate). Therefore, it’s imperative to consider how the power plant performs in that scenario. Improving the accuracy of P90 loss estimates with time-series modeling can reduce the uncertainty in reported energy estimates. This helps project owners minimize the financial and operational risk of their solar project.
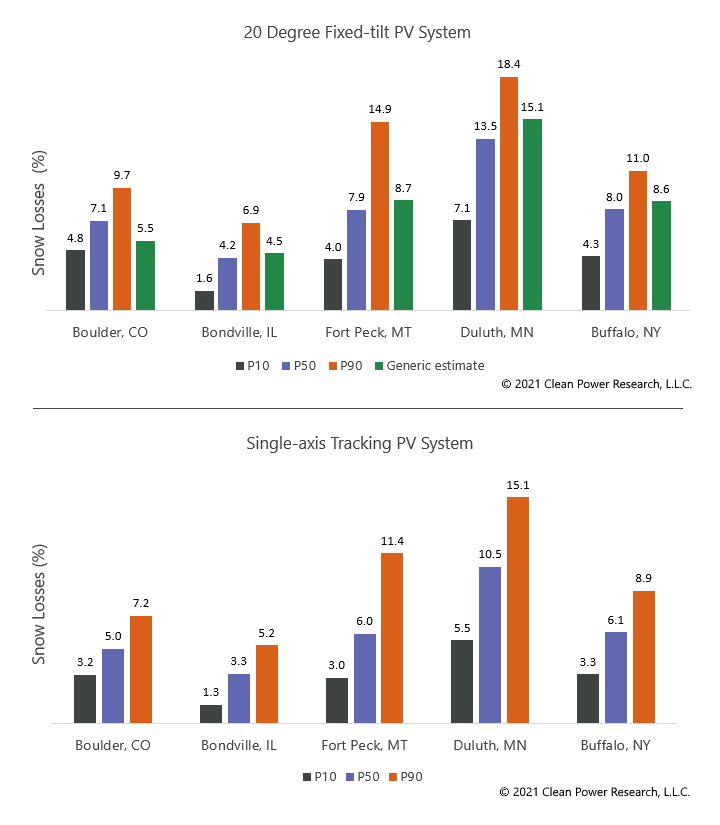
Figure 6: Snow Losses for a 20-degree Fixed-tilt and Single-axis Tracking PV System Using Generic and Specific Loss Approaches
References
1 Marion B, Schaefer R, Caine H, Sanchez G. 2013. Measured and Modeled Photovoltaic System Energy Losses from Snow for Colorado and Wisconsin Locations. Solar Energy, Volume 97: 112-121. DOI: 10.1016/j.solener.2013.07.029. Link
2 Ryberg DS, Freeman J. 2017. Integration, Validation, and Application of a PV Snow Coverage Model in SAM. NREL Technical Report. Link
3 Hourly snowfall above which snow coverage is set to the row’s slant height. Unit is centimeter per hour. SolarAnywhere uses a default value of 1.0 for this parameter.
4 Gun D, Kimball G, Anderson M. 2018. Dynamic Snow Loss Model and Validation. PVPMC 2018:7. Link
5 Townsend T, Powers L. 2011. Photovoltaics and snow: An update from two winters of measurements in the SIERRA. 37th IEEE Photovoltaic Specialists Conference (PVSC). DOI: 10.1109/PVSC.2011.6186627. Link.
On July 16, 2021, this page was updated using corrected snow depth data.